Understanding PSL Scores: A Comprehensive Guide
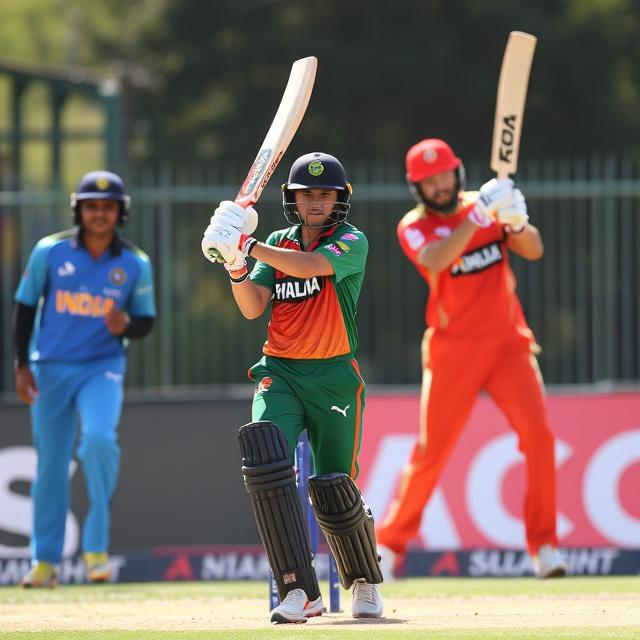
Decoding PSL Scores: What They Mean and How to Interpret Them
Ever wondered what those cryptic numbers represent in a PSL report? Understanding PSL scores is crucial for anyone working with or analyzing large language models (LLMs). This article will provide a comprehensive overview of what PSL scores signify and how you can interpret them effectively.
Unveiling the Secrets of PSL Scores: PSL, or perhaps more accurately, Prompt-Specific Language Model Performance, scores provide an insightful glimpse into a specific language model’s response capabilities when given a particular prompt. It’s not a measure of the overall quality, but rather how well a model excels at delivering an appropriate output for a particular prompt.
What Factors Influence PSL Scores?
Several factors contribute to a PSL score. These include the complexity of the prompt itself, the model’s training data, and the model’s architectural design. A high PSL score doesn’t inherently mean the model is better than others across all tasks; it just performs impressively when dealing with a certain prompt. For example, one model might excel at creative writing prompts, while another might dominate summarizing complex texts.
Think of it like a student’s exam performance. A high score on a math test doesn’t necessarily mean the student is an overall top performer; it indicates mastery of the mathematical concepts tested.
How to Interpret PSL Scores Effectively
To effectively interpret PSL scores, context is paramount. Review the specific prompt used to generate the score. What is the language of the prompt? What is the style? How was the expected output defined? Analyzing these aspects helps provide valuable insights.
Consider comparing PSL scores across different language models for the same prompt. This comparative analysis can reveal which model is best suited to the specific task. Don’t make decisions based on a single PSL figure—look for trends in a group of scores.
The Importance of EEAT in PSL Analysis
The EEAT (Expertise, Experience, Authoritativeness, Trustworthiness) principle is critical when reviewing PSL scores. If you’re researching language models for a specific application, ensure the sources you’re using are backed by reliable data and expertise within the field. Incorrect or misleading information can lead to flawed conclusions. A model’s PSL score should never be the only factor considered.
By understanding the factors that affect PSL scores, you can effectively analyze and interpret these results to identify the most suitable language models for specific tasks. Remember, PSL scores are just one piece of the puzzle when evaluating LLMs.
This article provides a starting point. Further research and experimentation are crucial for gaining a deeper understanding of these complex language models.